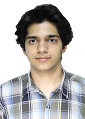
Mojtaba Hedayatpour
University of Regina, Canada
Title: Design and development of a learning-based human-analogous real-time control method with applications in industrial automation
Biography
Biography: Mojtaba Hedayatpour
Abstract
In the animal kingdom, humans learn by acting on their environment, observing the consequence or effect of their acts, and learning to adjust their actions accordingly over time to improve the output generated by their actions. They also learn to optimize their actions through reinforced learning. The ability of humans to optimize their behavior in natural systems can be expanded to that in man-made engineered systems as well. There is a world-wide effort towards developing control systems that are inspired from human intellect. This research is a timely response to this global need. The focus of this research is to develop a learning-based human-analogous control method that can be incorporated into the process of industrial automation in a short time and in a systematic way. This research addresses the design of Human Machine Interfaces (HMI) for re-enforced learning that can replace the time-consuming process of model-based control strategies currently exercised via the from-the-first-principle modeling paradigm. The real-time I/O data obtained within a Human-In-The-Loop (HITL) control system are used to: (1) optimize the design of the HMI, (2) optimize the learning curve associated with the trials via a consistency matrix, and (3) design optimal unmanned control strategies based on the most-consistent I/O data obtained via the real-time experiments in the aforementioned HITL simulator. Case studies are provided for a benchmark control problem, namely the servo control of a rotary actuator with unknown dynamics.